News
Real-time Waste Classification using Quasar
In a collaboration with VITO, Suez and Umicore and with support of EIT Raw Materials, we designed a waste classification algorithm which was fully implemented in Quasar. High-speed and real-time processing were part of the requirement, the conveyor belt rolls at a fast, industrial speed. Therefore, the sensors must be able to analyze the waste streams quickly enough, just like the software and algorithms have to process all measurements within a few seconds. The faster you can process data, the more waste you can characterize in a short time. Working faster also means quicker calculations, so we had to optimize the processing speed of the algorithms.
By using Quasar technology, the critical part of the processing chain could be off-loaded to the GPU, and a processing speed was obtained that is 133 times faster than a CPU-only implementation.
Read the full article here.
AutoSens 2020
We will be at AutoSens 2020 on Wed Nov 18, 4:55 PM – 5:20 PM GMT +0 / 5:55 PM – 6:20 PM CEST !
We will discuss some key programming principles for heterogeneous systems, in particular how to optimize across components (on the low-level image processing level and on the system level) to answer questions such as above. We present our own Quasar compiler and programming framework for this purpose. We will demonstrate our latest Radar-RGB sensor fusion results.
For more information: click here. See you there!
Autonomous vehicles making use of Quasar
Simultaneous localization and mapping (SLAM)
Martin Dimitrievski and his colleagues propose a novel real-time method for SLAM in autonomous vehicles. The environment is mapped using a probabilistic occupancy map model and EGO motion is estimated within the same environment by using a feedback loop. Input data is provided via a rotating laser scanner as 3D measurements of the current environment which are projected on the ground plane. The local ground plane is estimated in real-time from the actual point cloud data using a robust plane fitting scheme. Then the computed occupancy map is registered against the previous map in order to estimate the translation and rotation of the vehicle. Experimental results demonstrate that the method produces high quality occupancy maps and the measured translation and rotation errors of the trajectories are lower compared to other 6 degrees of freedom methods. The entire SLAM system runs on a mid-range GPU and keeps up with the data from the sensor which enables more computational power for the other tasks of the autonomous vehicle.
“Many of the Autonomous Vehicles sub-systems are massively parallel and this is where Quasar can speed things up. From pre-processing of LIDAR point cloud data to odometry, object detection, tracking and route planning, Quasar made all of these components possible to run on a mid-range GPU in real-time. When you are done prototyping, you can consult the profiler to easily spot any areas for improved execution of the code.” – ir. Martin Dimitrievski
Example: SLAM for autonomous vehicles
Reference
“Robust matching of occupancy maps for odometry in autonomous vehicles“; Martin Dimitrievski , David Van Hamme , Peter Veelaert, Wilfried Philips in
proceedings of Joint Conference on Computer Vision, Imaging and Computer Graphics Theory and Applications 2016 (VISIGRAPP).
Acknowledgements
The work was financially supported the Flanders Make ICON project 140647 “Environmental Modelling for automated Driving and Active Safety (EMDAS)”.
MRI reconstruction speedups up to x20 using GPUs
Magnetic Resonance Imaging (MRI)
MRI is a very powerful and safe medical diagnostic tool, but it is prohibitively expensive to use frequently. Hence, a technique that speeds up MRI acquisition would not only be helpful for patients, as it requires them to lie still for shorter periods of time, but it would also be of great benefit for doctors, as it leads to a higher patient throughput and a reduced susceptibility of the images to motion artifacts. Using Quasar, Jan Aelterman and his colleagues developed a reconstruction algorithm that handles acquisition speedup correctly.
Speeding up MRI is done by reducing the amount of acquired data. However, signal processing theory states it is impossible to do this beyond the Nyquist limit, without losing information. However, it is possible to only lose superfluous image information, this is called compressive sensing (CS). The danger is that that a naive reconstruction technique inadvertently corrupts an image while filling in lost information.
Proposed Technique
An MRI image is constructed from so called Fourier, or k-space coefficients. Due to acceleration techniques, the number of Fourier coefficients is less than the number of image pixels, resulting in an infinite number of possible images that would correspond with the acquired Fourier coefficients (an infinite number of ways to fill in the missing, superfluous information). Therefore, we impose an additional constraint: the reconstructed image is the one with the lowest number of shearlet coefficients possible. The shearlet transform can represent natural images with few coefficients, but not noise and corruptions. It is optimal in this respect. Hence, its use will force a noise – and corruption – free reconstruction. The optimization of the proposed problem requires iteratively applying the Non-uniform fast Fourier transform (NUFFT), which after profiling turns out to be a major bottleneck. By accelerating the NUFFT using the GPU we are able to gain significant speedups.
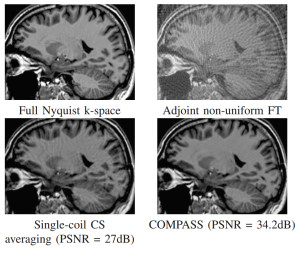
COMPASS reconstruction experiment using 4 acquisition coils and 10% of the Nyquist sampling rate on a k-space spiral.
Implementation was done using the new programming language Quasar, which allows for fast and hardware agnostic development, while still using the computation power of the GPU. Without requiring long development cycles, we were able to achieve speed-ups up to a factor 20 on an NVIDIA Geforce GTX 770. The speed-up achieved by the GPU acceleration opens the path for new and innovative research for MRI reconstruction: e.g. auto calibration, 3D reconstruction, advanced regularization parameters, etc.
“It took experts using CUDA/C++ three months to implement our MRI reconstruction algorithm; a developer using Quasar for the very first time achieved the same numerical results at the same computational performance in less than a single development week.” – Dr. ir. Jan Aelterman
Example
Reference
“COMPASS: a joint framework for parallel imaging and compressive sensing in MRI“; Jan Aelterman, Quang Luong, Bart Goossens, Aleksandra Pizurica, Wilfried Philips; in proceedings of IEEE International Conference on Image Processing ICIP(2010).
Quasar facilitates research on video analysis.
Optical Flow
Simon Donné and his colleagues achieved significant speed-ups for Optical Flow, a widely used video analysis method, thanks to the use of GPUs and Quasar. Tracking an object through time is often still a hard task for a computer. One option is to use optical flow, which finds correspondences between two image frames: which pixel moves where? In general, these techniques compare local neighbourhoods and exploit global information to estimate these correspondences. The authors presented their new approach at the conference for Advanced Concepts for Intelligent Vision Systems (ACIVS) in Catania, Italy.
“Thanks to the Quasar platform we have both a CPU and GPU implementation of the approach. Therefore we achieve a speed-up factor of more than 40 compared to the existing pixel-based method” – ir. Simon Donné
Example
Reference
“Fast and Robust Variational Optical Flow for High-Resolution Images using SLIC Superpixels“; Simon Donné, Jan Aelterman, Bart Goossens, Wilfried Philips in Lecture Notes in Computer Science, Advanced Concepts for Intelligent Vision Systems, 2015